The key components that encapsulate analytics exploration comprise data exploration, visualisation, different types of analytics (descriptive, diagnostic, exploratory, predictive), exploratory data analysis (EDA), statistical analysis, machine learning, big data exploration, interactive exploration and hypothesis testing. Embarking upon data exploration equates to immersing oneself deeply into the data's structure, quality, and inherent characteristics. This is accomplished through data profiling, summary statistics, and visual exploration methods.
Visualisation tools form a vital part of analytics exploration, utilising diagrams, graphs, dashboards and more to illuminate complex data patterns. They help interpret intricate details into comprehensible visual forms, enabling analysts and stakeholders to fully grasp the data.
Descriptive analytics refers to the process of summarising and interpreting historical data, offering insights about past events. This forms the foundation for subsequent explorations and analyses. In contrast, diagnostic analytics aims to understand why particular incidents took place, highlighting correlations and relationships within the data. It provokes a deeper examination of cause-and-effect relationships.
Exploratory Data Analysis (EDA) utilises a unique approach to examine data sets, deploying visual and statistical methods to summarise their chief characteristics. It involves creating visualisations, confirming assumptions, and discovering patterns. Statistical analysis techniques are employed to decipher relationships between variables, measure significance, and estimate uncertainties in the data.
Predictive analytics create models using historical data to predict future events or outcomes. Machine learning algorithms can enhance analytics exploration by identifying patterns or making forecasts. They train models on historical data, which are then applied to new, unseen data.
From a big data standpoint, analytics exploration entails dealing with large, complex datasets. It often requires distributed computing frameworks and specific tools for efficient data analysis. Interactive exploration allows analysts real-time interaction with the data, giving them the freedom to modify parameters and visualisations instantly to deepen their understanding of the information.
Hypothesis testing involves formulating hypotheses about the data, followed by utilising statistical tests to either confirm or refute these hypotheses. Analytics exploration is a cyclical process, constantly refining questions, adjusting analyses, and exploring various aspects to fully understand the data.
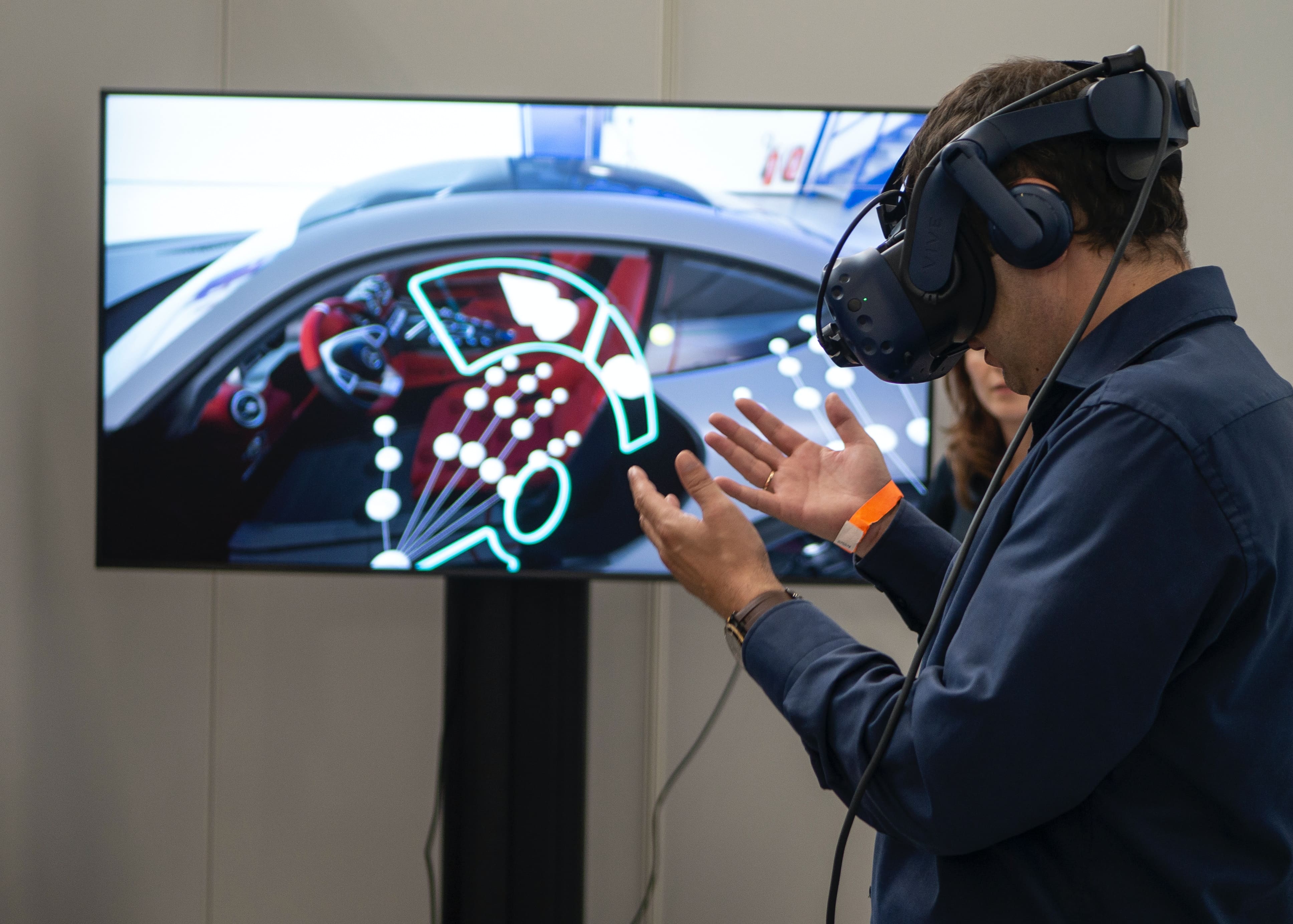
The insights discovered from analytics exploration can prove pivotal in strategic planning, decision-making, and streamlining business processes. Our Analytics Research team transforms raw data into actionable insights, empowering businesses to make informed decisions. With expertise in data collection, organisation, and interpretation, our analysts uncover crucial patterns and market shifts.
Using methodologies such as statistical scrutiny, data mining, and predictive analytics, we shed light on customer dynamics, industry trajectories, and performance indicators. What if we told you that you can consolidate your data to deliver high-precision analytics, regardless of where it resides, to unlock its potential value? Addressing your data confusion and converting it into intelligence equates to data-first modernisation, done wisely.
You might feel that data-driven modernisation is intimidating. However, it is possible to breakdown the journey into recognisable steps to simplify the process. Develop a common understanding of the role of legacy data platforms and a common language to define priorities.
Our Clients
BBC
Microsite for CBeebies Grown-Ups
Cisco
Website platform for Meraki Go
Harrods
Web platform and apps for luxury retail
Oakley
Gamification for European product launch
Pinterest Presents
An unparalleled virtual event, streamed live for a global audience
Volkswagen
Web platform and CRM for motor industry